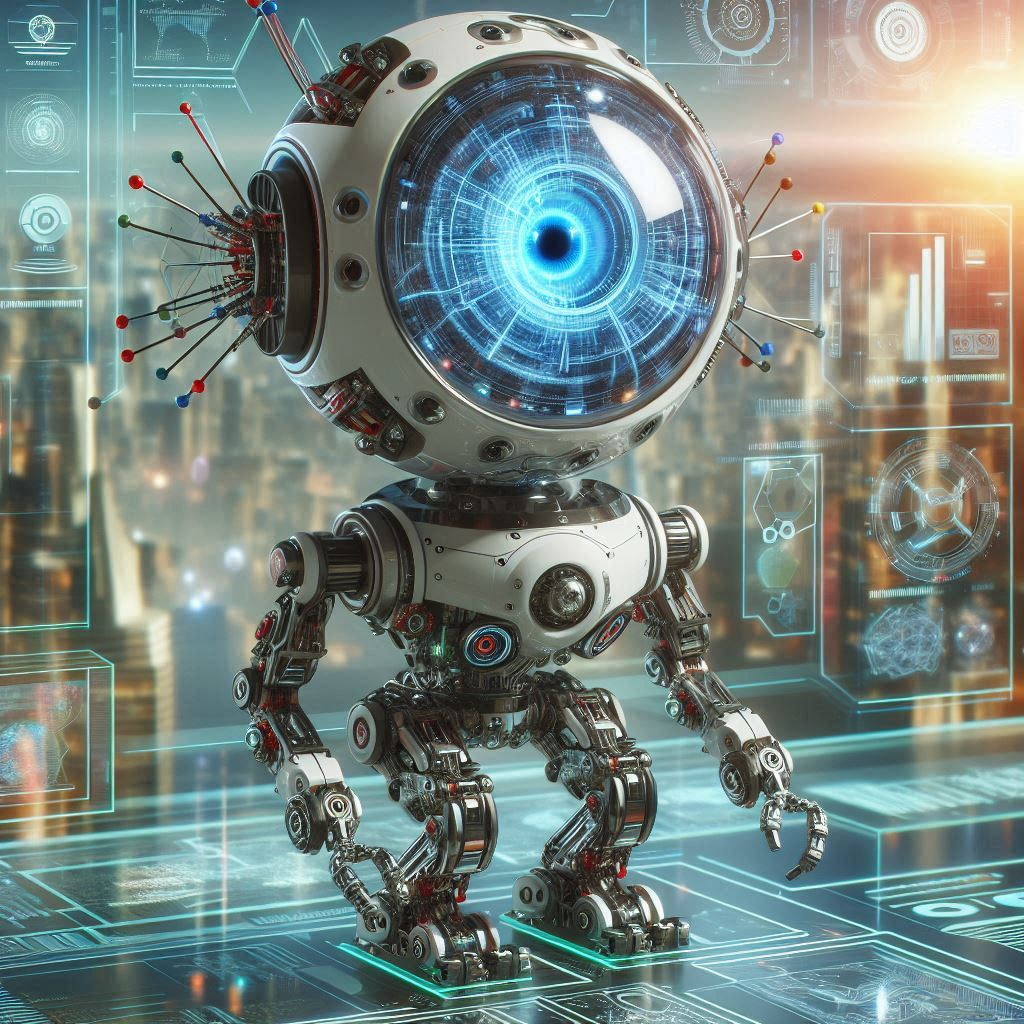
Principles of Autonomy: Action
Perception, Decision, and Action in Autonomous Robot Systems
Autonomous robot systems are perhaps one of the most revolutionary inventions of today, primarily designed to accomplish a desired task without human intervention, by observing their surrounding environments, making decisions and acting upon these decisions, through the core operating principles of perception, decision and action. This blog explores the basic components of autonomy: perception, decision and action, and the applications of autonomous robot systems.
Perception: Sensing and Understanding the Environment
Sensory Input
Autonomous robots have a number of sensors to collect information from the environment. Sensors include:
- Cameras: Capture visual information, essential for tasks like object detection and recognition.
- Light Detection and Ranging (LiDAR): A sensor that shoots laser pulses to map distances and generate three-dimensional models of the environment.
- Ultrasonic Sensors: Generate sound waves to detect objects, commonly used for proximity detectors and obstacle avoidance.
- Infrared Sensors: Detect heat and motion, useful for identifying living beings or heat-emitting objects.
- GPS (Global Positioning System): Provides geolocation and time information, crucial for outdoor navigation.
Data Processing
The raw data produced by sensors needs to be transformed to build up a coherent representation of the environment, by:
- Image Processing: Filtering out irrelevant information from visual data (e.g., filtering, edge detection, segmentation, etc).
- Sensor Fusion: Bringing together visual data from multiple sensors to create a more accurate and reliable representation, for example combining LiDAR and a camera.
- Point Cloud Processing: Used to convert LiDAR data into detailed 3D reconstructions of the surroundings.
Object Recognition
Autonomous robots need to recognize objects in their environment to interact with them, including perceiving and distinguishing between objects.
- Computer Vision: Computers perceive images and objects using algorithms that translate pixels into information. Examples include convolutional neural networks (CNNs) for the classification of images and for the detection of objects.
- Machine Learning: A process of training models on very large data sets to get better at recognizing objects over time.
Localization and Mapping
One of the most important is the Simultaneous Localization and Mapping (SLAM) problem. SLAM allows a robot to build a map of an unknown environment while at the same time tracking where in that map it is. Components of SLAM include:
- Feature Extraction: Identifying distinctive landmarks in the environment.
- Data Association: Matching observed features with previously detected ones.
- Map Update: Continuously updating the map as the robot explores new areas.
- Localization: Using the map to determine the robot's position and orientation.
Decision: Making Informed Choices
Decision-Making Frameworks
Autonomous robots use various frameworks for decision-making, each with its strengths:
- Rule-Based Systems: Operate based on predefined rules and logic, suitable for predictable environments.
- Machine Learning: Prediction and classification based on data-driven techniques. Examples include supervised learning, unsupervised learning, and reinforcement learning.
- Reinforcement Learning: Robots figure out problem-solving via trial and error, being rewarded or punished for their action. This method is useful in dynamic and uncertain environments.
Path Planning
The robot needs to decide how it should reach a destination: this is known as a path-planning problem. Two common robotic algorithms used for path planning are:
- A* Algorithm: Traverses the least-rewarded nodes first so that it finds the shortest path, given cost functions.
- Dijkstra’s Algorithm: Given a graph with nodes and weights, determines the shortest path between two nodes, while visiting all nodes exactly once.
- Rapidly exploring Random Trees (RRT): Efficiently explores large, complex spaces to find a feasible path.
Obstacle Avoidance
Real-time obstacle avoidance is essential for safe operation. Techniques include:
- Dynamic Window Approach (DWA): Checks for future velocities that would allow the robot to avoid obstacles while taking into account the robot’s dynamics.
- Potential Fields: The obstacles are tackled as repulsive forces, the goals as attractive, and a path that avoids pits and leads to the goal is found.
Behaviour Planning
Behaviour planning identifies actions based on the robot’s goals and the corresponding environmental context. This includes:
- Finite State Machines (FSMs): Define states and transitions based on specific conditions.
- Behaviour Trees: Behaviour Trees build on the idea of sequencing behaviours, allowing you to build complex behaviours as compositions of basic ones.
Action: Executing Decisions
Actuation Systems
The actuation system devices that allow the robot to move and manipulate objects. The components are:
- Motors: Provide rotational movement, used in wheels and joints.
- Servos: Offer precise control of angular position, essential for robotic arms and hands.
- Hydraulic and Pneumatic Actuators: Move quickly and smoothly, are very strong, and are found in larger robots and industrial use.
Control Systems
Control systems ensure that the robot's movements are precise and accurate. Key concepts include:
- Proportional-Integral-Derivative (PID) Controllers: Keep the desired positions or velocities by adjusting control input based on the feedback of errors.
- Model Predictive Control (MPC): Uses a model of robot dynamics to predict future states and optimise control actions.
Feedback Loops
Feedback loops are crucial for correcting actions and improving performance. These loops involve:
- Sensor Feedback: Continuously monitoring sensor data to detect deviations from desired behavior.
- Error Correction: Adjusting control inputs to minimize errors and achieve the desired outcome.
Robustness and Adaptability
Autonomous robots need to be robust and flexible to operate in uncertain environments. Targeted approaches include:
- Redundancy: Using multiple sensors and actuators to ensure continued operation if one component fails.
- Adaptive Control: Modification of control parameters in reaction to environmental or robot-internal changes.